- experiMENTAL
- Posts
- The five ways to measure Marketing ROI
The five ways to measure Marketing ROI
Here are the 5 tactics I’ve used to measure > $1.5B in Marketing spend and generate $180M+ in revenue for companies like Uber, DIRECTV, Otrium, and others.
👋 Hey, it’s Sundar! Thanks for reading experiMENTAL: my newsletter helping founders and marketers navigate the CRAZY world of consumer tech with secrets from 10+ years in Marketing at Uber & others.
In today’s newsletter, you’ll learn:
The 5 tactics
Marketing Science is the fun job of trying to prove and improve the ROI of Marketing campaigns.
Our sole reason for existence is for CMOs to come up with elaborate campaigns and tell us in the 11th hour that they want statistically accurate ROI calculations, so that they look good to the CEO.

I’m just kidding. Totally not triggered at all.
But seriously, I’ve been in Marketing Data Science for 10+ years and it is one of the most exciting jobs out there. Tests are hard to set up, problems are complex, and you’re working on things that touch the customer.
Below, I’ll share the 5 types of analysis I’ve used to measure > $1.5B in Marketing spend and generate $180M+ in revenue for companies like Uber, DIRECTV, Otrium, and others.

The types of analysis are plotted on a scale of 1 (least) to 5 (most) for both accuracy and complexity.
The goal is to be in the top right corner but as you’ll see that’s never the case.
Buckle up kids! Here we go

IYKYK.
Definitions
Complexity → The complexity of a methodology refers to how difficult it is to setup and analyze. There is an added layer of complexity when it comes to communicating results based on the methodology as well but I didn’t take that into the score.
Accuracy → The accuracy of a methodology refers to how statistically accurate it is and how much confidence you can place in the results.
Campaign → I’m assuming you understand what a campaign is but you may see it referred to here also as an intervention.
Metric of interest → The metric of interest aka primary metric is the metric you want to influence with your campaign.
Pre period → aka Pre is the period of time before a campaign starts. It’s usually defined in # of weeks.
Post period → aka Post is the period of time after a campaign starts. It includes the period of the campaign itself too.
Pre/Post
1/5 complexity, 1/5 accuracy
The Pre/Post methodology is named that because it compares a metric from the pre period of a campaign (Pre) to what happens in the post period (Post).
It’s a simple method that has been around since the dawn of time.
Example

Let’s look at the chart above.
In this example, they wanted to measure the impact of a test on “Mean Knowledge Score”.
They survey a group of students before the test (blue).
They survey a group of students after the test (red)
They compare the results.
The “Mean Knowledge Score” has gone up from before.
How to set it up
Pick your metric of interest
Understand how long your campaign is going to run
Create a baseline in the Pre period (usually the length of your campaign)
Launch your campaign
Calculate the metric of interest in the Post period
Compare the difference
Drawbacks
The biggest challenge is that it’s very hard to isolate the impact to your metric of interest from just your campaign.
In today’s world there are so many factors:
PR
Seasonality (the ultimate scapegoat)
Competition
Pricing changes
Product changes
Algorithm changes
Macro factors like war, economy, etc.
With the emergence of more digital channels and social media, your campaign and company can explode or implode within days.
You might think ”I’ll keep looking back longer in the Pre period to stabilize the data set”.
You’ll end up just spending more time explaining through factors.
It’s just really tough and when you present the analysis people from random teams will chirp in with “Have you thought about this”. You couldn’t have thought of everything.
When to use it
If you’re a startup that understands its seasonality and only has 1 to 2 channels without any other tools.
Even then, you have to make sure you’re not shipping any major product changes or increasing budgets in your other marketing channels.
If you don’t fit the criteria above, don’t use it.
Please.
Just please don’t use it.
Actually, I might just simplify to NEVER USE IT.
Diff-in-diff
2/5 complexity, 2/5 accuracy
Diff-in-diff stands for Differences-in-differences.
It is the slightly more mature and responsible version of a Pre/Post.
It has guardrails and an extra set of data that increases confidence.
It’s not complex and is more accurate but as you’ll see it has a lot of pitfalls.
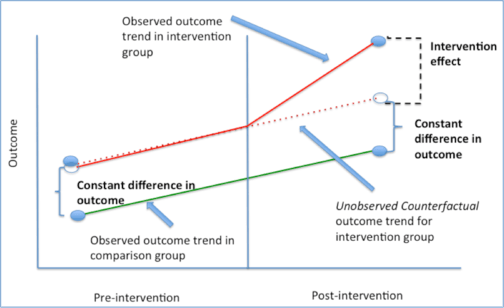
The methodology is the name and well explained in the chart above.
Example
You want to launch a campaign in Paris and are asked to estimate the impact.
For your business, London and Paris behave very similar.
You look at the 6 weeks before your planned campaign start and observe that the 2 cities act similarly and that the gap between London and Paris is fairly constant.
You then launch a campaign.
How to set it up
Start by identifying what your treatment group will be (likely a Geo otherwise you could A/B test)
Then identify another group that acts similarly in the Pre period
Measure the difference in the Pre period (difference # 1)
Then, monitor the relationship after the campaign starts
Measure their difference in the Post period (difference # 2)
Measure the difference in the differences (difference # 2 - difference # 1)
Step 6 is then your estimate for what the impact of your campaign is.
Drawbacks
Diff-in-diff requires a stable relationship between the 2 groups in both the pre period and the post period.
This means that all the challenges with Pre / Post also apply here.
Let’s revisit our Paris / London example.
Once you launched the campaign, you noticed a huge difference between London and Paris after the launch of your campaign.
Looks like your campaign is crushing it!
Except it’s July 2024 and a little thing called the Olympics is happening in Paris at the same time as your campaign.
Unfortunately, London doesn’t also have the Olympics going on.
The relationship between London and Paris have totally changed from the assumptions we were using in the pre period.
You can no longer use a Diff-in-diff methodology to estimate the impact.
This is an extreme example and any good Marketer should know the Olympics is coming up but it’s representative of all the hurdles you have to consider when using a Diff-in-diff methodology.
When to use it
If you’re a startup that understands its seasonality and has multiple cities, products, or other comparable factors that are big enough to be compared.
Even then, you have to make sure you’re not shipping any major product changes or increasing budgets in one group or another to impact the test.
Diff-in-diff is also a good methodology just to sense check your results but not as the primary tool for measurement.
It’s best for measuring offline channels and simpler tests.
Geo Testing
5/5 complexity, 4/5 accuracy
If Diff-in-diff and A/B testing had a baby, you would get Geo Testing.
Geo Testing is a like an A/B test but it splits up based on geography and not individual users.
However, it’s more like Diff-in-diff because it’s not perfect randomization.
You choose the control and treatment markets through a process called Market Matching that allows for you to control more factors.
Example
You’re a Paid Search manager owning the PPC campaigns for your business.
You want to know the incrementality of your campaigns.
Unfortunately, you can’t do a clean A/B test because Google doesn’t allow for it.
So, you opt for a Geo test.
You pick New York and Philadelphia as the cities you want to understand the impact of your testing. These are your treatment cities.
You then pick Chicago and Miami as your control cities.
You turn off your PPC campaigns in Chicago and Miami.
And then you compare between treatment and control.
This chart above is a good visualization.
Through the process of Market matching (explained below) the yellow phase shows that treatment and control act similarly in the Pre period.
You then use a validation period to confirm the relationship in the Pre period.
Then the campaign launches (which is the blue section) and you hope to see a difference.
How to set it up
Pick your treatment cities
Use a Market Matching methodology to find comparable control cities
Note: My former manager is well known in the Marketing Science industry and wrote a R package to help with finding control / treatment cities: Kim Larsen R Package
Use a period to validate your match before launch
Launch campaign
Evaluate difference
Drawbacks
The biggest challenge is the pre work and finding a set of cities / geos that act similar.
You would think it would be easy in a place like the US but let’s take an example from Uber:
Uber operates in 10K + cities. Within the US, it’s hundreds (maybe thousands) so on a high level finding comparable cities would be easy.
But, each city has different products, pricing, regulatory factors, etc.
So now you have to find cities that generally look a like from those factors or the geos you pick wouldn’t make sense.
In addition, there are often political considerations like “Do we really want to exclude this city from the test?”.
Everyone feels Fomo including geos excluded in a geo test.
These factors plus the coordination required to ensure a clean test in geos without contamination makes it a 5/5 in complexity.
When to use it
When you can’t do a classic A/B test.
Usually A/B experiments are run at the individual user and randomly assigned into treatment and control.
There are two situations where that’s not possible:
User level targeting is not possible
User level testing is not valid (Eg. a marketplace where it impacts the network)
This methodology is great for non digital marketing channels (TV, direct mail, offline billboards, etc.).
It’s also great for many of Google’s search driven products like Product Listing Ads, SEM, SEO, etc.).
Causal Inference with Propensity Matching
4/5 complexity, 4/5 accuracy
If you’re still here with me then we’re about to get real nerdy.

In an A/B test, we randomize the population before anything happens.
However, in many instances, we can’t run an A/B test for ethical, business, or logistical reasons.
So, we use a tool called propensity matching and we “randomize” it after.
Example
Uber has a loyalty program called Uber One.

They make a huge bet that it’s going to change the world.
But they don’t want to A/B test it because it’s weird for only some people to get access to a membership while others don’t.
More importantly , in marketplaces, it’ll impact the marketplace dynamic causing the whole test to not be independent.
CHAOS. So, Uber decides to roll Uber One.
A bunch of people sign up.
Most don’t.
Now, what’s the impact of Uber One?
Enter propensity matching.
We know the users that signed up for Uber One.
We know their demographic and behavioral information.
So, there’s probably a bunch of users who didn’t sign up for Uber one but “look” exactly like the ones that did.
Let’s compare apples to apples and only compare those that “look” like they should have bought Uber One and didn’t to those that “look” like they should have bought Uber One and did.
How to set it up
Identify the users that have taken your key action as “Treatment”
Identify demographic characteristics of the treatment that are important and might influence their decision (gender, age, wealth, riding behavior etc.)
Then use propensity matching to find “Control” users
Note: My former colleagues have designed a package just for this called CausalML.
Compare impact normalizing for propensity scores for Treatment and Control
Let’s look at the example chart above.
Before matching, treatment was overwhelmingly male while control was less so.
Already there’s a bias.
Then, we use propensity matching and compare similar populations.
Now, we see that the proportions are virtually identical.
We removed bias in our test that would otherwise force us to throw away any analysis.
Drawbacks
Like anything associated with using models and algorithms:
data quality
availability of data
legality / ethicality etc.
It’s also not an easy methodology to explain results.
When to use it
When you have individual data but can’t A/B test.
A/B Test (3/5 complexity, 5/5 accuracy)
The gold standard. The creme de la creme. The numero uno. The head honcho.

An A/B test is when a group of individual users are randomly split into two groups and shown two different experiences.
We then observe how one group performs vs the other group.
A/B tests are the most well know methodology and the most accurate.
Many tools have made them a lot easier to run making the complexity and set up quite low.
Example
You’re in charge of the email program at your company.
You have an idea for 2 different subject lines:
A: “Sundar has a decent newsletter”
B: “Check out why 300 founders read Sundar’s newsletter”
How do you know which one is better?
An A/B test 🙂 .
A random half of users will get A and a random half will get B.
The email content will be the same but just the subject line will be different.
You then send the emails and see which one has a better open rate.
Drawbacks
I honestly can’t think of any when done right.
The reasons companies don’t use A/B testing right is not the fault of the method.

From a measurement perspective, its the gold standard for a reason.
When to use it
Whenever you can.
Particularly efficient for email, push, and other owned channel marketing.
Recap
That’s it for this week. Some key takeaways:
Methodologies have tradeoffs in complexity and accuracy
Pre/Post and Diff-in-diff are not accurate but also not complex
Geo testing is great when you can’t A/B test at a user level
Propensity matching is great when you have user level data but cant test
A/B test is the 🥇
👍️ Loved it? 👎️ Hated it? Let me know!
Missed the last article?
Check it out: Quantify the health of your business
Reply